The External Relations Market Insight Team have been working in collaboration with the IT Innovation Team to develop new ways of analysing and understanding the large volumes of free text feedback that is generated by the National Student Survey (NSS).
Every year, final-year undergraduates are asked to complete the NSS, a survey which asks them about their experience of university. During the survey, they are asked to provide comments on the most positive and negative aspects of their university experience. Each year, this generates hundreds of thousands of words. This feedback contains important insights that the University can use to improve services and student experience.
Reading this many words and comments and analysing them for trends, sentiment, and key issues has historically been a time consuming and difficult process. People simply aren’t wired to read thousands of comments and perform detailed analysis on them, but machines can be.
Using AI and Natural Language Processing
Using the collective technical expertise and experience of reading thousands of historical student comments, the Market Insight and IT Innovation Teams decided to explore if it were possible to build an AI language model that could read feedback and categorise comments based the University’s goals and challenges. The idea was to teach AI to read the comments like a member of the team, but much quicker and in more detail than is normally possible.
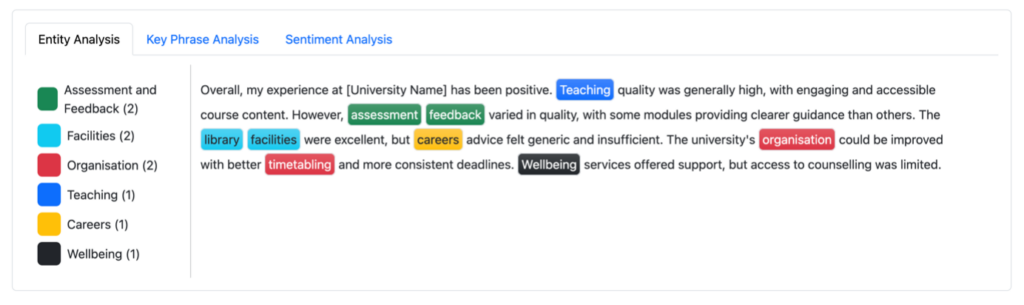
The model that was created was trained on ~1,000 historical comments and was designed to read and organise comments into the following categories:
- Assessment and Feedback
- Community
- Organisation
- Wellbeing
- Teaching
- Facilities
- Careers
The technology
Using the insights and expertise gained from previous years, the team developed a user-friendly web app that simplifies the process of analysing feedback from surveys. The solution enables people to use very powerful AI through a simple web interface, without needing to understand the complexities of AI.
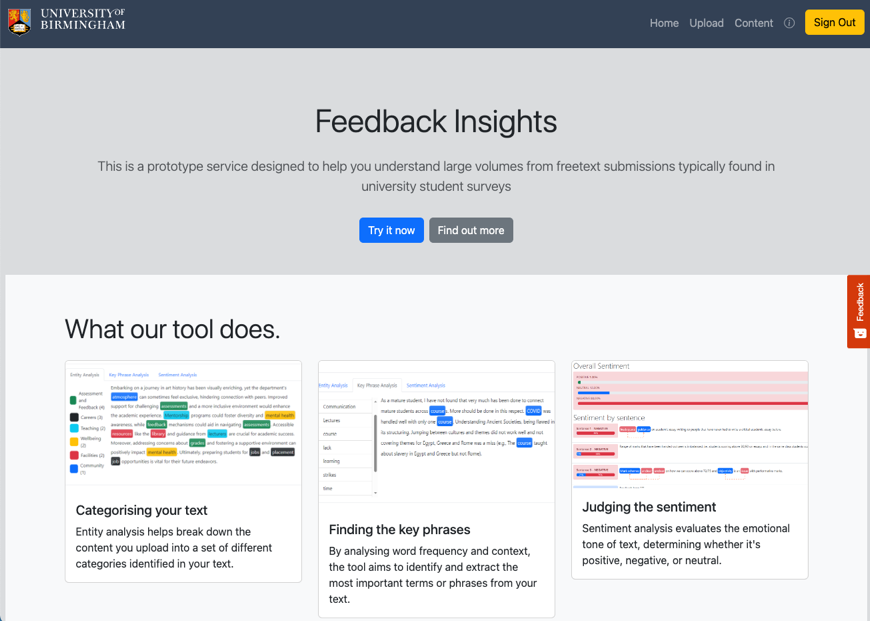
Figure 2 – A simple to use app that anyone can use.
The tool uses a branch of AI called Natural Language Processing (NLP) to perform categorisation of comments, key phrase analysis, and sentiment analysis. The technology can read and analyse 250 comments of student feedback in less than two minutes. This provides insights and data exports back to users in seconds and minutes, rather than days and weeks.

Figure 3 – Tool allows user to search and ask questions about the comments in minutes

How the technology performed during NSS
In previous years, it would normally have taken two people in Market Insight a whole day to read and redact the 5,000+ comments (that is without classifying the comments into themes). If further thematic analysis was needed, it would have taken a week or even longer to manually classify the comments and present them in a useable format for the Colleges.
This year, using the outputs from the Innovation Team’s web app, the Market Insight Team were able to produce an interactive Excel dashboard displaying comments by subject, programme, and demographics, filterable by theme and sentiment, within less than 2 days. Something of this scale would never have even been attempted in previous years due to the resource needed, but, without the technology, it would surely have taken the best part of a month to reproduce.
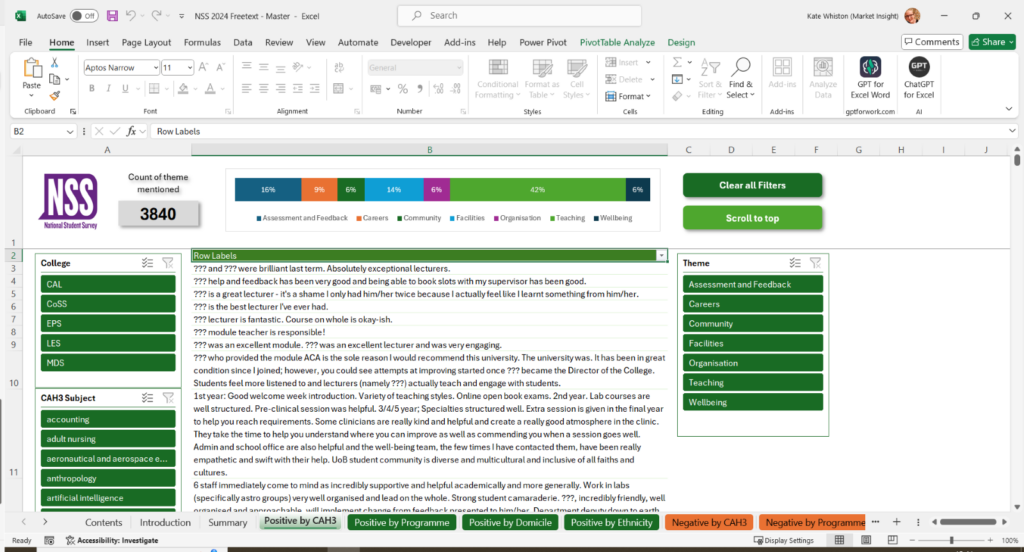
The time saved meant that Market Insight could invest greater effort into making the outputs as accessible and user-friendly as possible. The AI model was never designed to replace human interpretation – it is still essential that Colleges read all their comments! What the model did do, however, was allow Market Insight to present the rich freetext comments in an easily digestible format, in a fraction of the time, allowing Colleges faster access to the comments and make it easier for them to dig deeper into the detail.
The future
After this successful trial with NSS comments, we have also been testing our AI model’s performance using feedback from other surveys. Initial observations have found our NSS model relatively useful in other teaching and learning contexts, such as alternative undergraduate surveys, postgraduate surveys, and comments from students in Dubai. In the future, the ideal scenario would be to have specific models for each survey, tailored and trained on previous comments within their own context (i.e. language used, themes discussed, etc.). This is definitely possible with this technology and we hope to see more models being built in the not-too-distant future.